A comparative analysis of two significant policy documents, namely U.S. President Joe Biden's Executive Order on Safe, Secure, and Trustworthy Artificial Intelligence AI ("U.S. AI Executive Order") and the joint European Data Protection Supervisor & 45th Global Privacy Assembly Resolution on Generative Artificial Intelligence Systems ("Global GenAI Resolution"), underscores a fundamental truth:
AI does not introduce “New” legal challenges; rather, it sheds light on the challenges of upholding long-established "Old" legal principles in today’s AI-driven world. Conversely, the popularity of AI underscores the importance of revisiting two “Old” approaches to data protection to enable “New” AI capabilities in a lawful, ethical, and responsible manner that respects and upholds the integrity of fundamental personal rights while protecting enterprise trade secrets and intellectual property, namely:
AI does not introduce “New” legal challenges; rather, it sheds light on the challenges of upholding long-established "Old" legal principles in today’s AI-driven world. Conversely, the popularity of AI underscores the importance of revisiting two “Old” approaches to data protection to enable “New” AI capabilities in a lawful, ethical, and responsible manner that respects and upholds the integrity of fundamental personal rights while protecting enterprise trade secrets and intellectual property, namely:
- Anonymization of PII and Personal Data using Synthetic Data (as enabled by Anonos’ Data Embassy software) to support lawful and ethical AI model development and training;
- Statutory Pseudonymization of PII and Personal Data using controllably relinkable deidentified data (as enabled by Anonos’ Data Embassy software) to support lawful and ethical AI model use in production.
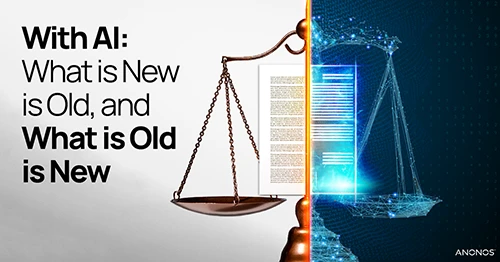
What is New is Old: Navigating AI's Legal Frontier
While AI has existed for several decades, it is only recently that “new” large language models, powered by generative pre-trained transformers, have been developed into user-friendly formats, seizing the attention of the broader population and increasingly fueling generative AI applications that impact people's daily lives.1 Within this setting, the U.S. AI Executive Order and the Global GenAI Resolution illuminate the pressing need to address re-identification risks and adapt regulatory frameworks. However, beneath the surface, we find a reaffirmation of enduring legal and ethical principles — “Old” principles that have withstood the test of time.
- Effectiveness of Protections: The U.S. AI Executive Order emphasizes the urgency of bolstering privacy protections in an age where AI facilitates the extraction and exploitation of personal data. Proposals range from supporting privacy-preserving techniques to advancing research in cryptographic tools. Similarly, the Global GenAI Resolution underscores the importance of weaving data protection and privacy into the fabric of AI systems. This entails integrating traditional cybersecurity controls with AI-specific safeguards and embracing privacy as the default option.
- Accuracy of Resulting Data: Both documents recognize the paramount need for accuracy in generative AI systems. The Global GenAI Resolution calls for developers to rely on reliable and representative data supported by robust data governance procedures and technical safeguards. The U.S. AI Executive Order seeks to harness AI's transformative potential while mitigating risks of inaccuracies. This includes enhancing AI's role in healthcare, education, and safety programs.
- Transparency of Actions: The Global GenAI Resolution mandates transparency measures for generative AI tools, ensuring openness in data collection and usage. Providers are tasked with informing deployers about data protection risks and facilitating external audits. To combat algorithmic discrimination, the U.S. AI Executive Order provides clear guidance, promotes best practices, and ensures fairness in various domains within the criminal justice system.
- Accountability: Both documents underscore the importance of accountability. The U.S. AI Executive Order calls for the development of standards, tools, and tests to ensure AI systems' safety and trustworthiness. The Global GenAI Resolution places the responsibility on developers, providers, and deployers to demonstrate compliance with national and international laws. Transparency in documenting AI models and their impact on data protection and privacy is paramount.
What is Old is New: Navigating Technical Safeguards for AI
While AI is evolving rapidly, some old-school techniques remain invaluable. In particular, two “Old” approaches—Anonymization and Pseudonymization—stand as opportunities to establish “New” standards for protecting data necessary for lawful and ethical AI.
Anonymization: Preserving Privacy and Promoting Innovation
- Anonymization removes or obscures personally identifying information (“PII”) and data that is linkable to data subjects’ identities (“Personal Data”) from AI models. This safeguard is critical in the AI realm, where large and diverse datasets are essential, but the use of data with PII/Personal Data can lead to privacy breaches and legal violations.
- Beyond legality, anonymization aligns with ethical imperatives. AI, including Generative AI, can mimic human behavior, making proper anonymization crucial to avoid unintended consequences.
- Anonymized data fosters innovation by providing researchers and developers access to valuable datasets while preserving individuals' privacy.
- Bias mitigation is another benefit of anonymization, reducing the risk of AI models perpetuating biases associated with sensitive attributes.
- Anonymization also promotes data sharing and collaboration among organizations and researchers, strengthening the AI ecosystem.
Synthetic Data: A Novel Approach to Anonymization
- Synthetic data offers a distinct solution that enables lawful and ethical AI via effective privacy protection. This technique first evaluates data to create mathematical models capturing statistical relationships within a dataset.
- These models generate entirely new records, preserving data's analytical utility while rendering them untraceable to the original records. Synthetic data is born anonymous, offering a fresh perspective on anonymization.
- In machine learning and AI, synthetic data provides quicker data access, retains utility, and carries a low risk of re-identification. Given these advantages, enterprises are adopting this technology for various purposes. For instance, a prominent German insurance company utilized Anonos' synthetic data to train a machine learning model on synthesized customer data for predictive analytics.
Pseudonymization: Balancing Accuracy and Privacy
What if relinking deidentified data back to identity is necessary to meet the requirements of a particular use case? For example, when using protected data for Retrieval Augments Generation, LLM fine-tuning, or submitting prompts to LLMs? While synthetic data makes de-identification irreversible, another technique called "Statutory Pseudonymization" - first defined under the GDPR and since adopted in many jurisdictions - can be used to preserve the accuracy and enable relinking that is limited to only authorized users under controlled conditions for use cases like these.
For those eager to delve deeper into this fascinating interplay of AI and law and to explore how Anonos' Data Embassy software is pivotal in this realm, we welcome your curiosity. Visit the Anonos website to request a demonstration and join a community at the forefront of redefining data protection for the AI age. Also, read the IDC report: Variant Twins: The Key to Safely Leveraging Data for AI for more information on how Anonos Data Embassy software creates Variant Twins that combine the best of Statutory Pseudonymization and Synthetic Data for AI.
- Statutory Pseudonymization is an advanced form of pseudonymization that transforms data to prevent re-identification by anyone other than the data controller, all without sacrificing accuracy or utility.
- Statutory Pseudonymization sets itself apart by requiring demonstrating protection for all of a data set, not individual fields, defense against singling-out attacks, dynamism in pseudonyms, selective non-algorithmic lookup tables, and controlled re-linkability.
-
Anonos Variant Twins enable Statutory Pseudonymization for using and fine-tuning AI models using controllably relinkable deidentified data to ensure both the accuracy and privacy of AI models using production data is maintained.
- For example, after an offshore third party trains an AI model to identify the attributes of qualified prospects for a new financial services offering, a Statutorily Pseudonymized version of production data can be created to run through the model. The results of running the Statutorily Pseudonymized production data through the model can then be made available to parties authorized to reverse the protections to reveal the identities of qualified prospects so that they can receive an offer for the new service.
For those eager to delve deeper into this fascinating interplay of AI and law and to explore how Anonos' Data Embassy software is pivotal in this realm, we welcome your curiosity. Visit the Anonos website to request a demonstration and join a community at the forefront of redefining data protection for the AI age. Also, read the IDC report: Variant Twins: The Key to Safely Leveraging Data for AI for more information on how Anonos Data Embassy software creates Variant Twins that combine the best of Statutory Pseudonymization and Synthetic Data for AI.
1. See International Association of Privacy Professionals (IAPP) article: Performant risk mitigation for AI and LLMs