In the evolving cybersecurity landscape, the CIA Triad and the Zero Trust security model have been considered the gold standard for protecting organizational assets by those responsible for safeguarding sensitive data and systems against increasingly sophisticated cyber threats.
However, as we pivot towards artificial intelligence (AI) to drive innovation and efficiency, these traditional security frameworks face new challenges that stem from the need for AI systems to access and process vast amounts of data, often in conflict with the stringent controls imposed by conventional Zero Trust security models.
Dive into this guide to understand how Zero Trust Data™ can increase security and augment your overall Zero Trust architecture. Discover how solutions that protect data in use enable organizations to boost security measures and facilitate access to and utilization of high-quality data effectively.
However, as we pivot towards artificial intelligence (AI) to drive innovation and efficiency, these traditional security frameworks face new challenges that stem from the need for AI systems to access and process vast amounts of data, often in conflict with the stringent controls imposed by conventional Zero Trust security models.
Dive into this guide to understand how Zero Trust Data™ can increase security and augment your overall Zero Trust architecture. Discover how solutions that protect data in use enable organizations to boost security measures and facilitate access to and utilization of high-quality data effectively.
Key Takeaways
-
Zero Trust Architecture emphasizes least-privilege access, rigorous authentication, and continuous monitoring.
-
While effective in conventional IT settings, this approach encounters obstacles with AI initiatives due to AI's extensive data access and processing requirements, which clash with traditional security constraints.
-
Access controls, pivotal to Zero Trust, are not entirely sufficient. They protect the perimeter but do not fully secure data in its most vulnerable state - when it is being used.
-
Zero Trust Data involves integrating AI with Zero Trust principles with a data-centric security approach, focusing on safeguarding the data itself.
- Anonos Zero Trust Data aligns security with innovation, ensuring a framework that facilitates data access and compliance.
Understanding the CIA Triad and the Zero Trust Architecture
The CIA Triad is a cornerstone of information security, embodying the three most crucial principles that define security measures and policies within organizations. These principles are confidentiality, integrity, and availability:
The central concept of Zero Trust revolves around the method of access control, the main principle being the least-privilege access (POLP) - controlling the access rights of users based on their roles within the organization and determining and restricting the data they can access.
Essentially, users and devices are only granted access to the resources required to carry out their duties, reducing the risk of unauthorized access and lateral movement within the network.
The Evolution of Zero Trust
The concept of “Zero Trust” appeared in 2010, introduced by a Forrester Research Inc. analyst. Google’s announcement of implementing Zero Trust security in its network further fueled the interest among the tech community.
In 2019, Gartner, a global research and advisory firm, recognized the significance of Zero Trust security access. It was identified as a key component of secure access service edge (SASE) solutions. As the age of IoT and digital transformation unfolded, the need to validate each access became crucial, leading to the widespread adoption of Zero Trust security models around the globe.
-
Confidentiality: Ensuring that sensitive information is accessible only to authorized individuals.
-
Integrity: Maintaining the accuracy and reliability of data.
- Availability: Guaranteeing that information and resources are accessible when needed.
The central concept of Zero Trust revolves around the method of access control, the main principle being the least-privilege access (POLP) - controlling the access rights of users based on their roles within the organization and determining and restricting the data they can access.
Essentially, users and devices are only granted access to the resources required to carry out their duties, reducing the risk of unauthorized access and lateral movement within the network.
The Evolution of Zero Trust
The concept of “Zero Trust” appeared in 2010, introduced by a Forrester Research Inc. analyst. Google’s announcement of implementing Zero Trust security in its network further fueled the interest among the tech community.
In 2019, Gartner, a global research and advisory firm, recognized the significance of Zero Trust security access. It was identified as a key component of secure access service edge (SASE) solutions. As the age of IoT and digital transformation unfolded, the need to validate each access became crucial, leading to the widespread adoption of Zero Trust security models around the globe.
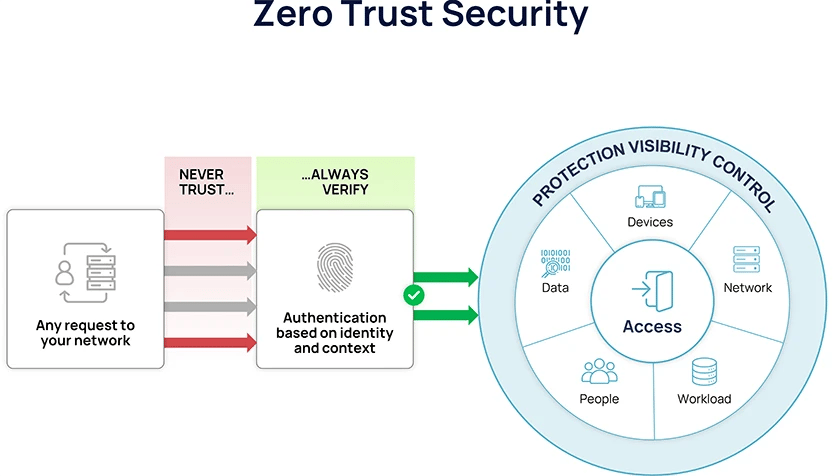
An illustration of the Zero Trust security, Privacy Affairs, 2023
Key Components of Zero Trust Architecture
In the architecture of Zero Trust, Identity and Access Management (IAM) holds a central role. Authentication mechanisms for access requests, such as two-factor (2FA) and multifactor authentication (MFA), are integral components of a zero-trust strategy, ensuring that each access request is authenticated before granting access based on user identity. Authorization is applied to every access request to ensure access is granted only to the necessary resources.
Microsegmentation is another critical aspect of Zero Trust Architecture. By creating discrete zones of network resources, microsegmentation allows IT to contain potential threats and prevent their lateral spread throughout the enterprise.
Microsegmentation is utilized in Zero Trust to enforce policies and restrict access, thereby implementing the principle of least privilege and reducing lateral movement within the system.
Continuous monitoring of user behavior and device health is essential to Zero Trust Architecture. It enables organizations to:
In the architecture of Zero Trust, Identity and Access Management (IAM) holds a central role. Authentication mechanisms for access requests, such as two-factor (2FA) and multifactor authentication (MFA), are integral components of a zero-trust strategy, ensuring that each access request is authenticated before granting access based on user identity. Authorization is applied to every access request to ensure access is granted only to the necessary resources.
Microsegmentation is another critical aspect of Zero Trust Architecture. By creating discrete zones of network resources, microsegmentation allows IT to contain potential threats and prevent their lateral spread throughout the enterprise.
Microsegmentation is utilized in Zero Trust to enforce policies and restrict access, thereby implementing the principle of least privilege and reducing lateral movement within the system.
Continuous monitoring of user behavior and device health is essential to Zero Trust Architecture. It enables organizations to:
-
Proactively detect and respond to potential threats
-
Ensure that the security posture remains robust and up-to-date in the face of ever-changing threat landscapes
-
Consistently assess security controls and make adjustments as needed
- Maintain a strong security posture and protect against cyberattacks
The Bottlenecks Caused by Traditional Zero Trust Architecture in AI and Digital Transformation Initiatives
The integration of Zero Trust Architecture in AI and digital transformation initiatives presents a paradox.
While Zero Trust is designed to enhance security in traditional IT environments through rigorous access controls and continuous risk assessment, its principles often collide with the dynamic requirements of AI-driven initiatives.
This clash primarily arises from AI's inherent need to access and process vast amounts of data, a necessity that traditional security models, including Zero Trust, can inadvertently stifle due to their stringent controls.
At the core of Zero Trust Architecture is enforcing access controls based on various contextual factors, such as user identity, device health, and data classification. This model operates on the "least privilege" principle, ensuring users are granted only the minimum level of access necessary for their job functions.
While this approach effectively safeguards resources at the gateway, it encounters limitations once data is in use - when it is most valuable and, concurrently, most vulnerable.
At the core of Zero Trust Architecture is enforcing access controls based on various contextual factors, such as user identity, device health, and data classification. This model operates on the "least privilege" principle, ensuring users are granted only the minimum level of access necessary for their job functions.
While this approach effectively safeguards resources at the gateway, it encounters limitations once data is in use - when it is most valuable and, concurrently, most vulnerable.