Understanding the differences between pseudonymization vs anonymization can be tricky. There are both legal and practical distinctions between the two, and each is suited to different types of data processing use cases.
But fear not. After reading this article, you’ll clearly distinguish these terms and know how to use each method practically in your business cases.
You’ll learn how to get the most out of each method, lawfully share data, conduct analytics, reduce data privacy risks, and ensure compliance with regulations.
And if you’re busy and just want to get a grasp of the difference between pseudonymization and anonymization, we share it right below.
Let’s first take a quick look at what data is being protected with these techniques.
But fear not. After reading this article, you’ll clearly distinguish these terms and know how to use each method practically in your business cases.
You’ll learn how to get the most out of each method, lawfully share data, conduct analytics, reduce data privacy risks, and ensure compliance with regulations.
And if you’re busy and just want to get a grasp of the difference between pseudonymization and anonymization, we share it right below.
Let’s first take a quick look at what data is being protected with these techniques.
What are PII, PID, and Personal Data
Understanding the different types of personal information is important for considering what you are protecting and why. Below, you’ll see what type of information falls into buckets of Personally Identifiable Information (PII), Personal Identifiers (PID), and Personal Data.
According to the National Institute of Standards and Technology (NIST):
According to the National Institute of Standards and Technology (NIST):
-
Personal Data is any information relating to an individual that could re-identify them, including direct identifiers, indirect identifiers, attributes, and other characteristics that could be used to relink to identity, including information related to a person’s physical, physiological, mental, economic, cultural or social identity. It is a much broader category than PII or PID.
-
Personally Identifiable Information (PII): Any representation of information that permits the identity of an individual to whom the information applies to be reasonably inferred by either direct or indirect means. All PII is Personal Data, but not all Personal Data is PII.
PII can be any information that lets you trace and identify an individual. This can be full name, address, passport number, email, credit card numbers, date of birth, telephone number, login details, and many more.
- Personal Identifiers (PID) are a subset of PII data elements that identify a unique individual and can permit another person to “assume” an individual's identity without their knowledge or consent. PIDs are driver's license numbers, passport numbers, biometric records, etc.
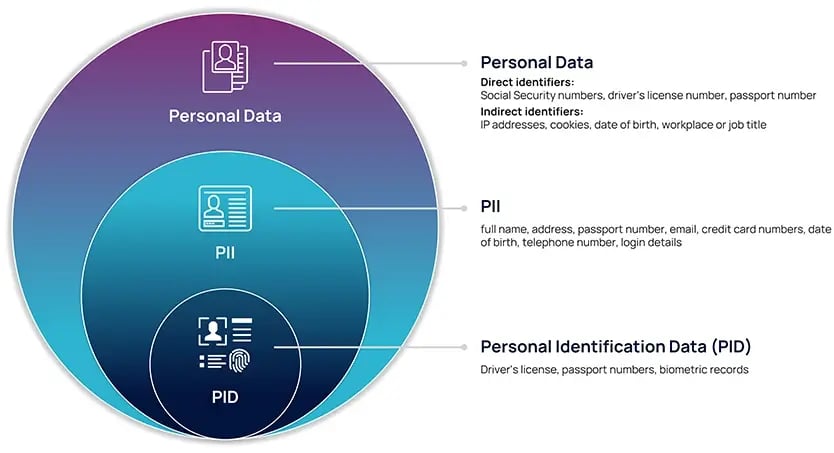
Now that you understand what PII, PID, and Personal Data are, let’s jump to the topic of anonymization and pseudonymization.
Knowing whether data is pseudonymized or anonymized is important. This is because truly anonymized data is exempt from the jurisdiction of the GDPR, while pseudonymized data is not. They are also useful for different use cases.
Let’s first take a look at pseudonymization.
Knowing whether data is pseudonymized or anonymized is important. This is because truly anonymized data is exempt from the jurisdiction of the GDPR, while pseudonymized data is not. They are also useful for different use cases.
Let’s first take a look at pseudonymization.
What is Pseudonymization and How Does Statutory Pseudonymization Differ?
Before the GDPR, pseudonymization was considered to be a technique. It was often thought of as interchangeable with masking or tokenization.
Pseudonymization was considered to be one method of de-identifying data, where the de-identification process could be reversed. It was viewed as a data protection technique primarily applied to the protection of direct identifiers.
When the GDPR came into force, pseudonymization took on a new meaning (one defined by law).
Pseudonymization under the GDPR has a legal definition that sets a much stricter standard than other data protection methods like masking and tokenization. The GDPR definition of pseudonymization is what we refer to when we use the term “Statutory Pseudonymization.”
Pseudonymization was considered to be one method of de-identifying data, where the de-identification process could be reversed. It was viewed as a data protection technique primarily applied to the protection of direct identifiers.
When the GDPR came into force, pseudonymization took on a new meaning (one defined by law).
What is Different About Statutory Pseudonymization?
Pseudonymization under the GDPR has a legal definition that sets a much stricter standard than other data protection methods like masking and tokenization. The GDPR definition of pseudonymization is what we refer to when we use the term “Statutory Pseudonymization.”
The GDPR defines pseudonymization as “the processing of personal data in such a manner that the personal data can no longer be attributed to a specific data subject without the use of additional information, provided that such additional information is kept separately and is subject to technical and organizational measures to ensure that the personal data are not attributed to an identified or identifiable natural person.”
Statutory Pseudonymization establishes a legal standard or an “outcome” to reach. You must adhere to this standard if you want to be compliant with the GDPR when using pseudonymization.
This legal standard goes beyond the use of one data protection technique or the other, and you may need to use multiple techniques to achieve it. Such a standard means that businesses must process personal data in such a way that it can’t be re-linked back to specific individuals without the use of additional, separately stored information.
Let’s take a look at the 5 specific elements that are necessary for this legal standard to be met.
Statutory Pseudonymization requires five different elements. This is an approach that goes beyond the pre-GDPR understanding of pseudonymization. It requires:
This legal standard goes beyond the use of one data protection technique or the other, and you may need to use multiple techniques to achieve it. Such a standard means that businesses must process personal data in such a way that it can’t be re-linked back to specific individuals without the use of additional, separately stored information.
Let’s take a look at the 5 specific elements that are necessary for this legal standard to be met.
5 Elements of Statutory Pseudonymization You Should Know About
Statutory Pseudonymization requires five different elements. This is an approach that goes beyond the pre-GDPR understanding of pseudonymization. It requires:
-
Protection of all data elements: This includes both direct and indirect identifiers.
-
Protection against singling-out attacks: Using K-anonymity and/or aggregation.
-
Use of dynamism: Using different tokens at different times for different purposes, and at different locations. This makes re-linking to identity much more difficult from a technological perspective.
-
Inclusion of non-algorithmic lookup tables: This alleviates some of the vulnerability of cryptographic techniques.
- Controlled re-linkability: The data controller must hold source data separately. They must also protect it with technological and organizational measures.
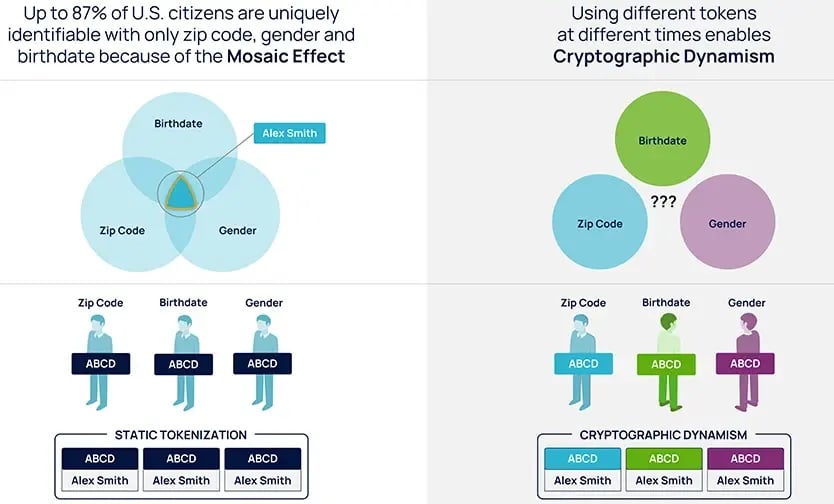
Static tokenization (left) vs dynamic tokenization (right)
In addition, Statutory Pseudonymization does not only protect direct identifiers (i.e. “obvious” personal information) but also indirect identifiers.
Indirect identifiers are information that does not relate to an individual explicitly, but can be connected with the individual in some way. This means that when you combine different data sets with information known to be tangentially related to an individual, you can in some cases use a combination of that data to re-identify them.
Since Statutory Pseudonymization provides a much higher standard of protection than basic tokenization and masking, your organization can reap a number of business and legal benefits by using it.
Statutory Pseudonymization offers further benefits for your business. These benefits include:
Statutory Pseudonymization can also be used in other industries such as insurance and banking. The most typical use cases include fraud detection, analytics, and risk assessment. For these use cases, pseudonymization protects sensitive insurance customer information, while retaining data utility.
Despite the many positive aspects of pseudonymization, there are some elements to bear in mind. For example:
There are also numerous legal benefits that come with Statutory Pseudonymization.
Business Benefits of Statutory Pseudonymization
Statutory Pseudonymization offers further benefits for your business. These benefits include:
-
Economies of scale: When data is properly protected, your business can use cloud-based infrastructure (IaaS and PaaS). You can also upload data to the cloud without fear of exposing sensitive data.
-
Faster project approvals: With scalable and predictable data protection controls, projects can be approved faster. This is because your legal and privacy teams can have more certainty about whether your projects are compliant. Pre-configured pseudonymization controls can also streamline similar data use cases, as many of the protection approaches remain the same.
-
Expanded projects and supply chains: With greater levels of predictable data protection, you can engage in more data sharing. This means your business can obtain more data from a wider variety of sources, enriching your data sets. This can be useful for expanding and improving use cases such as analytics, ML, AI, and monetization.
- Speed to insight: Statutory Pseudonymization does not reduce utility in the way that some masking techniques do. This allows you to get relevant information and results quickly so that you can focus on business outcomes.
Statutory Pseudonymization can also be used in other industries such as insurance and banking. The most typical use cases include fraud detection, analytics, and risk assessment. For these use cases, pseudonymization protects sensitive insurance customer information, while retaining data utility.
Despite the many positive aspects of pseudonymization, there are some elements to bear in mind. For example:
-
Remaining re-identification risk: There is no risk-free technique. With every approach, there is always a risk of re-identification that remains, and it is important to assess these risks and reduce them wherever possible. This is because there is always room for human error in implementing a technique, as well as sophisticated attacks against privacy on a continual basis.
-
Potential reduced utility compared to cleartext: Depending on which combination of specific techniques you use to achieve Statutory Pseudonymization, there may be some loss of utility. However, with Anonos’ approach to Statutory Pseudonymization, there is no loss of utility compared to cleartext.
-
Cost of implementation: All data protection techniques have set-up costs, and may need experts to help you implement them. Statutory Pseudonymization techniques are no exception. Like other data protection methods, it requires experts to ensure that it has been properly achieved.
- Remains personal data: Data that has been Statutorily Pseudonymized is still personal data under the GDPR. This means the law still sets out certain requirements for it. However, the GDPR also provides statutorily recognized expanded use rights for data that has been pseudonymized. The requirements and benefits that apply will depend on individual situations.
Legal Benefits of Statutory Pseudonymization
There are also numerous legal benefits that come with Statutory Pseudonymization.
-
Surveillance-proof processing: Pseudonymization is one of the approaches noted by the European Data Protection Board as enabling the transfer of data assets internationally in compliance with Schrems II and the U.S. Cloud Act.
-
Lawful processing: By meeting the standard of Statutory Pseudonymization, you can overcome the shortcomings of processing data based on consent and contract. This means you can carry out data processing under legitimate interest grounds. This is particularly useful for advanced analytics, artificial intelligence (AI), and machine learning (ML).
-
Breach-, ransomware- and quantum-resistant data processing: Statutory Pseudonymization de-risks data at rest, in transit, and in use. Sensitive details are obscured with Statutory Pseudonymization, which reduces attack surface area.
- Data supply chain defensibility: When data is protected with Statutory Pseudonymization, everyone in the data supply chain is protected against joint and several liability for data sharing, combining, and processing.
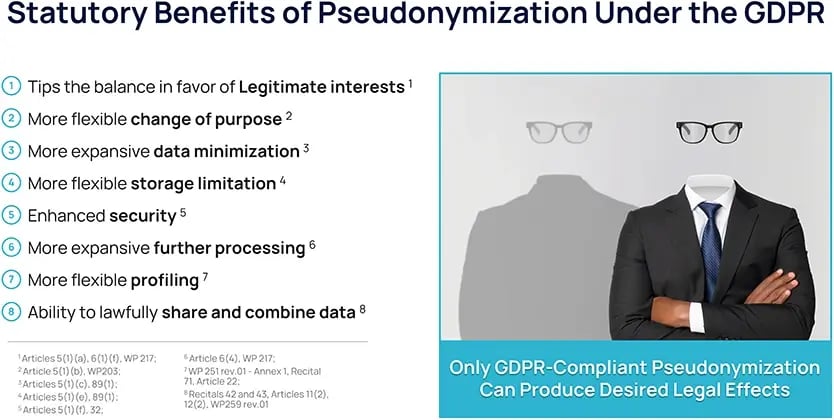
All of these benefits make Statutory Pseudonymization an attractive choice for enterprises. If you’re still wondering how you might use it in practice, let’s take a quick look at one example of what you might want re-linking for.
What is Statutory Pseudonymization Useful For?
Statutory Pseudonymization is useful when the identity of the data subject is still relevant for the use case.
This is often the case with medical and health records because the identity data of patients needs to remain connected with their records.
In addition, health professionals often share data between themselves, in the process of providing coordinated treatment. Anonymizing or aggregating medical data could strip away vital information necessary for patient care.
Thus, Statutory Pseudonymization is a valuable standard to meet, enabling secure data sharing while protecting patient identities. Importantly, the de-identified information can be re-associated with actual patient identities only by authorized individuals who have access to the separately stored additional information.
This approach is often used in health contexts globally. For example, Statutory Pseudonymization is similar to the most effective means of “de-identification” under the Health Insurance Portability and Accountability Act (HIPAA) in the US. One important note is that Statutory Pseudonymization actually allows greater utility and a higher level of privacy than what is mandated under HIPAA.
This is because HIPAA requires that certain identifiers be removed from records (called the “Safe Harbor” method), leading to loss of utility in some contexts and reduced privacy in others (when identifiers are left unprotected). HIPAA does not use the same terms of “pseudonymization” or “anonymization” as the GDPR, but it’s important to understand how these different terms are related to each other, especially if you are operating internationally.
We’ll get into the details of HIPAA and data protection laws from other countries later on.
It’s important to remember: While Statutory Pseudonymization has a number of benefits, it may not be the right choice for all use cases.
In certain situations, such as statistics and general analytics, re-linking to identity is not necessary or desirable. In these cases, anonymization, including the production of synthetic data, may be a better choice. While both methods intend to protect personal data, they do it differently.
Let’s take a look at anonymization in more detail.
In addition, health professionals often share data between themselves, in the process of providing coordinated treatment. Anonymizing or aggregating medical data could strip away vital information necessary for patient care.
Thus, Statutory Pseudonymization is a valuable standard to meet, enabling secure data sharing while protecting patient identities. Importantly, the de-identified information can be re-associated with actual patient identities only by authorized individuals who have access to the separately stored additional information.
This approach is often used in health contexts globally. For example, Statutory Pseudonymization is similar to the most effective means of “de-identification” under the Health Insurance Portability and Accountability Act (HIPAA) in the US. One important note is that Statutory Pseudonymization actually allows greater utility and a higher level of privacy than what is mandated under HIPAA.
This is because HIPAA requires that certain identifiers be removed from records (called the “Safe Harbor” method), leading to loss of utility in some contexts and reduced privacy in others (when identifiers are left unprotected). HIPAA does not use the same terms of “pseudonymization” or “anonymization” as the GDPR, but it’s important to understand how these different terms are related to each other, especially if you are operating internationally.
We’ll get into the details of HIPAA and data protection laws from other countries later on.
It’s important to remember: While Statutory Pseudonymization has a number of benefits, it may not be the right choice for all use cases.
In certain situations, such as statistics and general analytics, re-linking to identity is not necessary or desirable. In these cases, anonymization, including the production of synthetic data, may be a better choice. While both methods intend to protect personal data, they do it differently.
Let’s take a look at anonymization in more detail.