Audience Question
[00:50:55] In my background, I've done a couple of things. So, I'll ask two questions. One, I used to work as a privacy officer for an entity that looked into health systems that collected cancer research data, and one of the big issues was the longitudinal data for population surveillance studies and ethnicity in this case for that site comes up for that, but I'm also engaged now with some other players in the field that are starting to do - I don't know if you've heard these phrases - self-sovereign identity and various things like that. So, if you get a situation where self-sovereign identity takes off and you’ve got an otherwise cryptographically unique relationship so each individual has actually unique presentations with the various backend data stores, does that give you any problems?
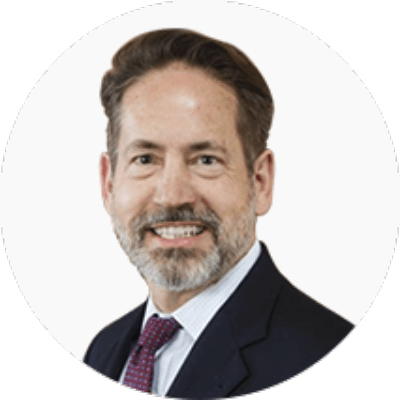
Gary LaFever (Anonos)
[00:51:45] So, a couple of things. First off, to go to your point indirectly and not go to it directly,, I'm actually flying first thing tomorrow to Oslo meeting with the Norwegian Data Protection Authority at the request of a life insurance company. Here's their concern. They were told by their technology team and not their CPO that they have to delete all the data when somebody leaves the insurance company. So, their concern is what happens when somebody comes back later to either ask for coverage or there's a client that they have no history whatsoever. And so, we're going together to the Data Protection Authority to discuss that this has societal impact. And so, what you can do, if you think of what a Variant Twin represents - a Variant Twin represents a non-identifying version of data with the ability to re-link back to the original data. You can sever the linkages and give the key that includes the linkages and the original data to the data subject. So, if they ever do come back, you actually can realign all of that.
[00:52:43] And so, the first thing I want to go to is the fact that there are impacts of some of these laws that if you don't think through can actually have negative impacts on the data subjects and society as a whole. Secondly, with regard to that specific question that you asked, the easiest way to think of the way that Anonos does what it does and people may well be familiar with the example that's often cited that you can get from the US Department of Census three data sets. People within the US residences and the last census by zip code, by age, and by gender. Each of those is anonymous according to US definitions of the term because people's names aren’t in it. The problem is my name was replaced in each three of those datasets by the same token.
[00:53:25] And if you combine those three datasets, it's been proven that you can re-identify up to 87% of the US population by name simply because I was given the ABCD in the first one, ABCD in the second one, and ABCD in the third. The easiest way to think of the easy implementation of our technology is what if I’m ABCD on the first one, Q99 in the third, and DDID in the second? The reality is each of those datasets is still accurate. You can't figure out who anybody is and any of them by themselves. And unless you have access to and permission to see the mapping table and overall key, you don't know that ABCD equals 1234 equals DDID. So, yes, you can in fact cover that thanks. Allison, how about you?
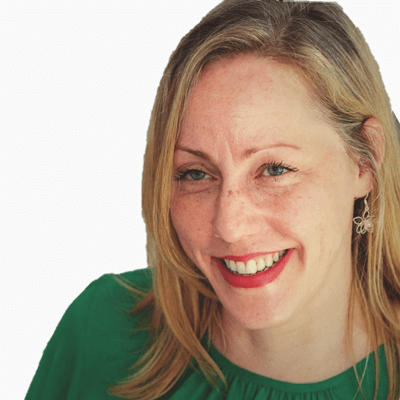
Dr. Allison Knight (University of Southampton)
[00:54:08] So, in my language and from where I come from, I think what you're talking about is data stores, so it’s this concept that you can actually give people to control the linkages in particular in identity assurance testing and I think that's actually possible using this same type of technology. So, actually, the government is coming up with something called Verify, which is a UK identity assurance scheme and it's really based on this model that you're using trusted third-party intermediary. So, use that trust level. Why do we need to know exactly who we're transacting with? You know, it's like having a credit card. Why does it have our names on it anyway? Why isn't it Mickey Mouse? So, why is that important to know? So, I think, yes, you're absolutely right. There is potential. This is a field of research, but how are we going to do it? Those principles that Gary and I found at that meeting of minds, I think, that's the key for the future way in which we’re going to do this stuff.
Audience Question
[00:55:05] So, I haven't read your paper and I'm just going off of what you said and I'm going to ask you a question if I'm interpreting correctly because I didn't see the definition of Variant Twins. Maybe you had one up there, but I didn't see it. So, it sounds to me like you're having temporary tokens that represent k-anonymous and l-diverse subsets of your entire set. Would that be an accurate description?
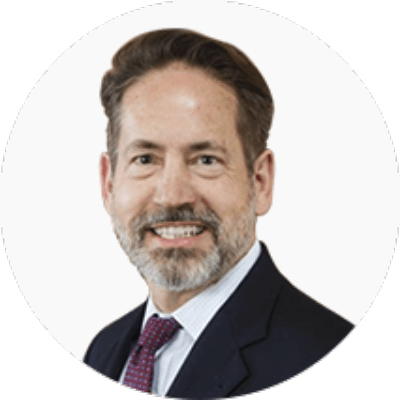
Gary LaFever (Anonos)
[00:55:34] Correct and then you're maintaining the mapping between them. Absolutely. You clearly know what you're talking about. Any other questions?
Audience Question
[00:55:40] So, the organization I work for supplies products and services direct into the NHS. Three separate types of business. So, there’s very little personal identifiable information in there. The second one is for staffing so putting the temp staff from agencies into trusts, and then the third portion is staff flow. So, you may have a temp worker logging in on one trust who has the skills and attributes to be able to fill the vacancies within other trusts for various different reasons. So, there are privacy impact assessments being conducted within the data processing of grievances created that’s going to be a set of anonymised data and we would only receive the anonymised data for the temp employee analytics. When we stop doing staff flow, so one employee is moving to a different trust, we need the un-anonymised data. The trusts are actually the data controller. We’re the data processor. So, there’s been a complete change of what that use of the data is going to be for. We would’ve originally received the data either from the trust or from an agency that's anonymised but then during that person’s employment they have provided us with all of the things that then identity that person as ABCD123. I guess the question is: How does that set and how do you get around that because there’s this massive data creep but the only way that we can do business is by there being the data that is accepted by all parties that this is going to happen, but there’s no documentation to actually say we should have that. There is no processing agreement as all of the agreements from the agencies and from the trusts directing the data subject. There is no agreement direct with us as a processor.
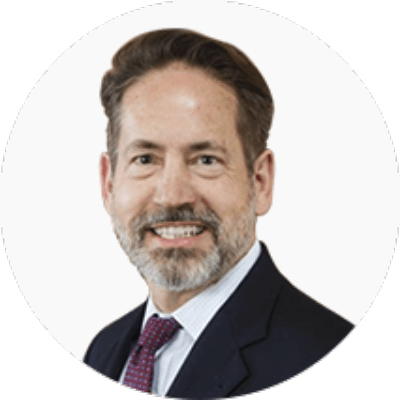
Gary LaFever (Anonos)
[00:58:34] So, Alison, do you want to take that one first?
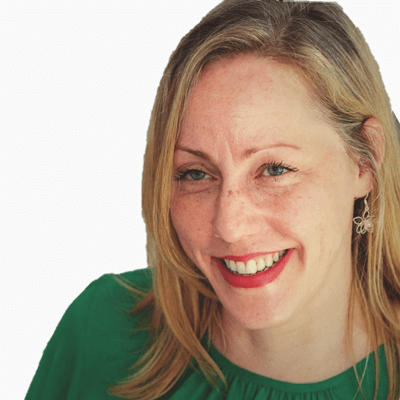
Dr. Allison Knight (University of Southampton)
[00:58:36] Well, whenever anyone ever says that the agreement is data is anonymised, my hair straightens up into the air. Part of the problem is that what does anonymisation even mean in particular under the existing regime? You know, traditionally, it's been touted sometimes where anonymous data is that there's no risk of re-identification. But of course we're not living in that world anymore where re-identification is a risk. It's a long tail of risk. So, I guess without getting into the details here, I’d ask how was it anonymised? But let's put that to one side. I think function creep or you said data creep so once you have identifiability - and I mean the GDPR doesn’t say that you can’t have some residual re-identifiable risk. What it says is that it depends on the means reasonably likely by the organization or perhaps the third party in certain circumstances to re-identify.
[00:59:35] So, I think you would have to sit down and try to work out what those consequences are. But really from a pragmatic point of view, I think you're right that at that point you're already there. You've already got the risk of it's personal data. So, you already have to be thinking about whether you'll comply. And this is a problem when it's all about terminology and it's about - once we get a late stage, we're left with a bit of a mess. How much better is if we could - how are going to define anonymisation process or Pseudonymisation as the ideal when we want to keep those individual data points. That's why we use the word Pseudonymisation. We want the individual data point so we can do things with it. We're not talking about advocating and taking away. So, I guess there were a lot of questions that you need to go through. But the next time, yes, you're right, it needs to be in a data processing agreement and then it's actually thinking about these things from the start. You know, I'm in a similar boat. The University gets information from other sources. Of course, there’s this question about transparency. At what point does the individual need to know what you're doing with their data as the subsequent point? But I would say fundamentally, first of all, you need to have that secure flow under Data Protection by Design to get you onto that right footing going forward.
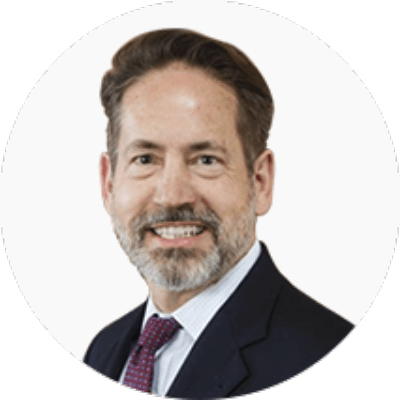
Gary LaFever (Anonos)
[01:00:50] So, I was on a video conference call yesterday. And without consciously doing it, the person on the other side used the term “anonymisation” and I went like this as if I was warding off some kind of Dracula or a demon. And the reason is, I think the word anonymisation gives us a false sense of security for all the reasons that Allison said, and Pseudonymisation is hard to say and it’s harder to spell. Is it an S? Is it a Z? And by the way, it’s not pseudo-anonymisation because that word doesn't even make sense. But the good thing about Pseudonymisation and I have been mentioning this - the definition of Pseudonymisation under the GDPR has never existed before. It literally says: “I need to separate the information value of data from the means to re-identify. There must be additional information necessary to reunite those and that must be kept under controlled conditions and only provided to those people who have the authorized parties.
[01:01:49] That's why if I replace a value with the same token every time, I don't need additional information. I can draw those correlations without anything. That's why to me - to us - static tokenisation does not satisfy the definition of Pseudonymization, and Pseudonymization is not required anywhere under the GDPR. It's never required. It is encouraged 13 times and there are significant benefits of using it. So, what I would say to you is first, don't use the A word. Use the P word right and then think about it. If you can show that technologically the data itself has been protected so that when it comes back and you really do have to do the re-linking that it requires additional technology and the data is only available to select people. That and a good privacy impact assessment and you should probably be okay, but I would avoid the A word because I really think it gets people in trouble and I would actually look for the technical measures.
Audience Question
[01:02:47] So, this A word is a stipulation from the data controller within their processing agreements you will only receive anonymised information. It can’t be anonymised information.
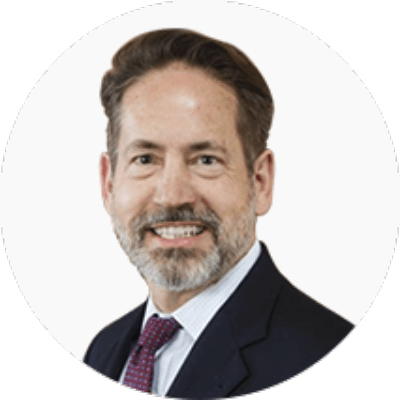
Gary LaFever (Anonos)
[01:03:11] I think it's Article 4 (5) of the GDPR. I’ll send it to him and just say: “Read the definition of Pseudonymisation.” Any other questions? We have a little bit more time.
Audience Question
[01:03:17] I was thinking if a data processor posts data that belongs to a controller and then wants to do product development through analytics, would they rather rely and say that this data is anonymous so GDPR doesn't apply after applying strong Pseudonymisation or that they actually become a controller and then the data subject right is documented?
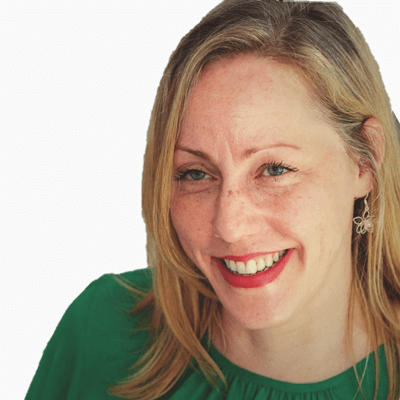
Dr. Allison Knight (University of Southampton)
[01:03:44] I mean, this is a tension that's throughout. You know, as in are people going to put all the eggs in one basket and say it’s anonymised, which we don't like that word, but anonymous for the purpose of GDPR, which means that you've got the Recital 26 which means that if you can't reasonably by the means likely you can't re-identify that person then it's non-personal data. But the problem with the dynamic view is that that means reasonably likely in the perspective - the how, the from whose perspective, and in what ways? I mean, even identifiability can be defined in different ways. It's very difficult to put your hand down. So, we love it. So, that's what you would do if you're trying to do analytics. You would handle the data in a pseudonymised form at the least. I mean, if you can take away all the individual data points and achieve your own, great. Do that.
[01:04:33] But if you can't, at the very least pseudonymise it so you've got a good argument with the regulator if any problem that it's non-personal data. But we're in a regime and era now where you can’t assume and put your eggs in a basket because if things change it could be treated. So, you need to contract to say that it is personal data. Whether they then become controllers is actually a slightly different issue. It really depends on what they're doing. That relationship could be. I mean, in the Data Pitch Project, we've talked a lot about that, but I would say that's slightly a separate issue from whether it's personal data or not. It's really who has the means and the purposes and who's driving this relationship and always seeks some legal advice around that.
Audience Question
[01:05:14] To your point as well, I think you touched on it a number of times in your presentation and I think it can't be understated. Is the importance of the controlled sharing because the minute you - and I always tell my clients this in presentations as well to never just release a dataset you think is anonymised or it doesn't have any personal data into the wild because, as you mentioned, it's dynamic and we're regenerating a thousand points of light and a million points of light in petabytes. So, I think in response to that, you're always going to have a contract and technical and other organizational measures that controls how it's going to be used and requires them to play within that little sandbox. And if they want to make any other changes, then they have to come back and you have to do the new risk assessment to see if it can be re-identified again after in light of the changing environment.
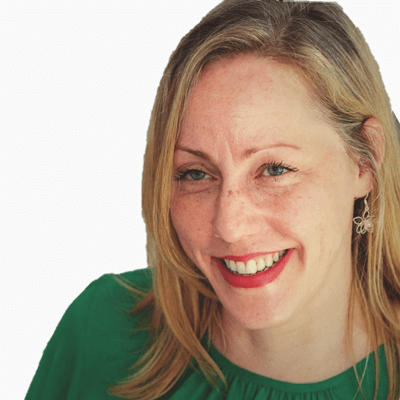
Dr. Allison Knight (University of Southampton)
[01:06:01] Yeah. That's absolutely right. So, break it down into time periods as your purpose changes as the data or what we call the data environment changes. And you're actually right as well. You can't just say technology was there. It's a combination of legal. No one would share that data with that data legacy agreement. We're not going to get rid of lawyers and I can say that because I'm a lawyer. Fortunately, maybe in 20 years’ time. But you know you've got to have the right thing and the organizational - so this actually sits on top of what we've been learning about today about the GDPR organizational, the accountability, the keeping records, the getting lawyers involved without feeling like the legalease is overwhelming us and there are too many agreements, and then as well as that we understand the framework and we use technology to do the work for us and we don't have to do so much work. So, it's a culminated suit of armor that we're putting on there and understanding that is key.
Audience Question
[01:06:55] And I'm just wondering and back to you, with that in mind then, you provide the technology and the tool, but do you also sort of caution your clients that this is not the magic button?
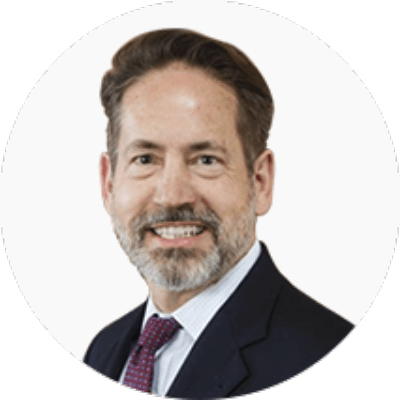
Gary LaFever (Anonos)
[01:07:02] It is not a silver bullet. In fact, what it's about is really - we were at the IAPP Global Conference in DC a couple of weeks ago and someone came up to her booth and they said: “My company just spent over $50 billion to buy a healthcare company. I have 12 people in my compliance department. And before the acquisition, we had 700 data scientists. After the acquisition, we're going to have 1400 data scientists.” Guess how many people are going to be in the compliance department? 12. And so, their question to us was: “What do you actually do?” And the answer when you cut through the k-anonymity and the l-diversity, which is important, is we programmatically enforce your policies. It's not a silver bullet, but you can now create policies that are technologically enforced. They’re your policies. Not ours. It's your data. Not ours.
[01:07:55] And so, to us, Data Protection by Design and by Default is all about just that. No, it doesn't replace lawyers. It makes them superheroes. You see the T-shirt that we’re giving out, right? Because it programmatically gives you the technical ability to enforce the rules that you determined makes sense. And so, 8 weeks from now when the Chief Data Officer comes in and says: “Can I still use my data?” Hopefully, you have this and you say: “Yes, you can. But guess what? You can do anything you used to do before but you have to do it differently and I'm here to help you figure out how to do that.” So, data analytics, artificial intelligence, machine learning, digital transformation, it's actually even more enabled under the GDPR. If you embrace the technical and organizational measures and the safeguards it requires and the data governance. I mean, it’s really what it's about. Any last questions? I think we have just a moment or two. Yes, please?
Audience Question
[01:09:00] I’m Lisa Tang. Sometimes when you have the government agencies - so, if you want to claim like VT for example and then they want proof, evidence, customer list or attendee list or something, can you then challenge them to say you don't really need this information and you just need to know the number of people attending the event so that you can then playback the VT. Could you then use this technology to say: “I'm going to give you this.” But it doesn't show you the personal data? And that you really need to see that they can have the key to it and that will only be given to a specific case work because if you give them the list, it could be shared amongst other people in the organization.
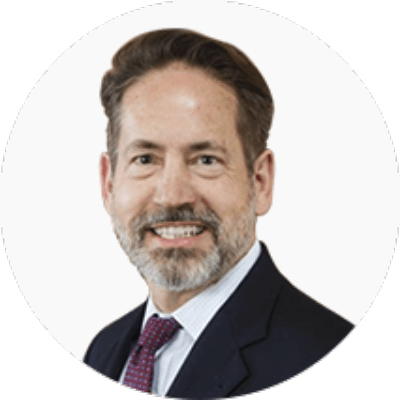
Gary LaFever (Anonos)
[01:09:45] So, what you just described is what I think we've all hoped surveillance becomes where surveillance is applied at the pattern matching level and not the individual identity level and only when abnormal or dangerous patterns are observed that you can pierce through to get identity. So, whether the government would accept that is another question. But I like to think that in time as people become more familiar with this and I'll speak to you - the debate will be what's the appropriate level of k-anonymity. If we ever get to that point, k-anonymity basically means there's at least a K number of people in an equivalence class. Typically, it’s held to be five, which means that there are at least five people that can be identically described in the same category. The risk of re-identifying them is no greater than 20% with any one of them.
[01:10:35] So, if you could say: “Look, I had 10 cohorts and there were five in each, which means I had 50 people.” I had 50 people and yes I have the data to show the five and each of those. If they would take that, actually that would be a great result because you do have the data that supports it in a fine grain or identifying manner, but it would only be pierced when justified. So, as odd as that sounds, my hope is someday we're arguing as to what is the appropriate level of k-anonymity. That would mean we've really advanced the point to where objective criteria used by regulators and governmental agencies will determine if we’re managing risk. Well, thank you very much. We appreciate the time.